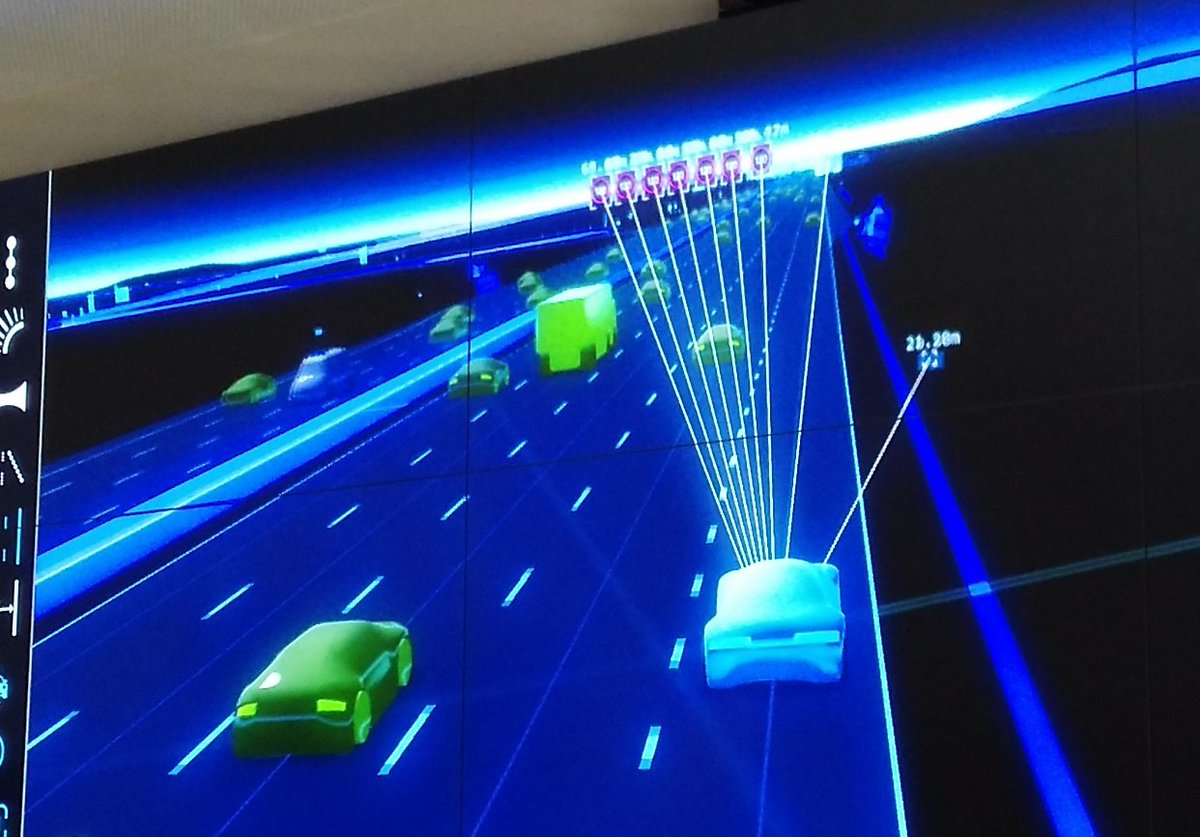
A startup with a billion dollar asset. This is how HERE’s new CEO Edzard Overbeek describes the location services company that is making a striking pitch for being the third major digital mapping and location platform alongside Google and Apple.
HERE has had an interesting recent history. Originally NAVTEQ, one of the major cross-world road network databases, used by various “sat nav” systems, it was bought by Nokia and became Nokia Maps, before being rebranded as Ovi Maps. Nokia then sold its phone business to Microsoft – but as the latter already had Bing Maps, the digital mapping business was spun off into a new unit and sold to a consortium of German car companies. At the time, this perhaps seemed a surprising new set of owners but it has quite quickly become obvious – with self-driving car technology suddenly seemingly closer on the horizon, the need to have a global, highly precise digital map of the world’s streets is suddenly incredibly important – the aforementioned billion dollar asset. Google has been building it up from its initial, low-precision mapping, using its fleet of LIDAR mapping cars, and Apple has been doing the same, arguably starting from an even worse base. HERE has arrived in the space with the highest quality start, having been based on a digital map that is over 20 years old.
The insideHERE Event
HERE was kind enough to invite me to an event, insideHERE, at their European headquarters in the heart of Berlin, for demonstrations of their portfolio, using some of the platforms used recently at MWC, CES and the other major trade exhibitions in the technology and mobile space. They also discussed a few “under the hood” features, and what they are working on right now.
There were three themes, reflecting the three main segments of digital mapping at the moment – business, consumer and auto. A cancelled flight at very short notice (thanks for nothing, Norwegian!) meant that I arrived in Berlin late and so missed the first two. The first can be summarised with the HERE Reality Lens Lens product which provides high quality asset and street furniture mapping for the use and management by local authorities, and the second is encompassed by the HERE mobile app digital app, which occupies the same space as Apple Maps and Google Maps app, aiming to displace these on their respective platforms. This is a challenge of course, as the existing apps are pretty good, so HERE’s unique selling point is that they are designed for offline from the ground up (Google Maps offers this on a slightly more restricted basis, but HERE will be available in offline mode for an area, as soon as you initially load it up online.) Reality Lens and the HERE Offline Maps app are nice pieces of technology that utilise data from HERE’s car data gathering options and make it accessible to public sector and consumer users respectively, but it was clear, both from HERE’s new owners and the comparative length of time used during the day, that HERE Auto is the key sector for the company now.
Geodemographics
HERE have developed geodemographic profiles for car users (drivers/passengers), based on surveys in the USA, South Korea and Germany. Using cluster analysis of the results, they have identified six characteristic types of users, based on how they use cars and other transport options, day to day:
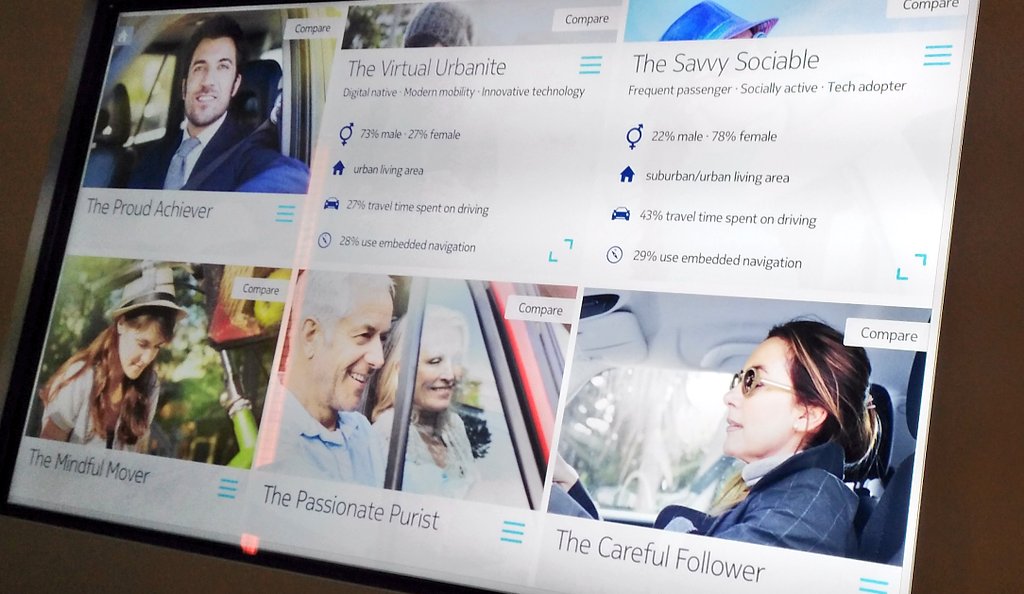
Autonomous Navigation Data
Here’s a visualisation of the datasets that HERE use for self-driving cars. These are datasets designed for machines, not people, and the maps of the datasets, shown here, show the breadth and detail of the information used by self-driving cars to determine road information:
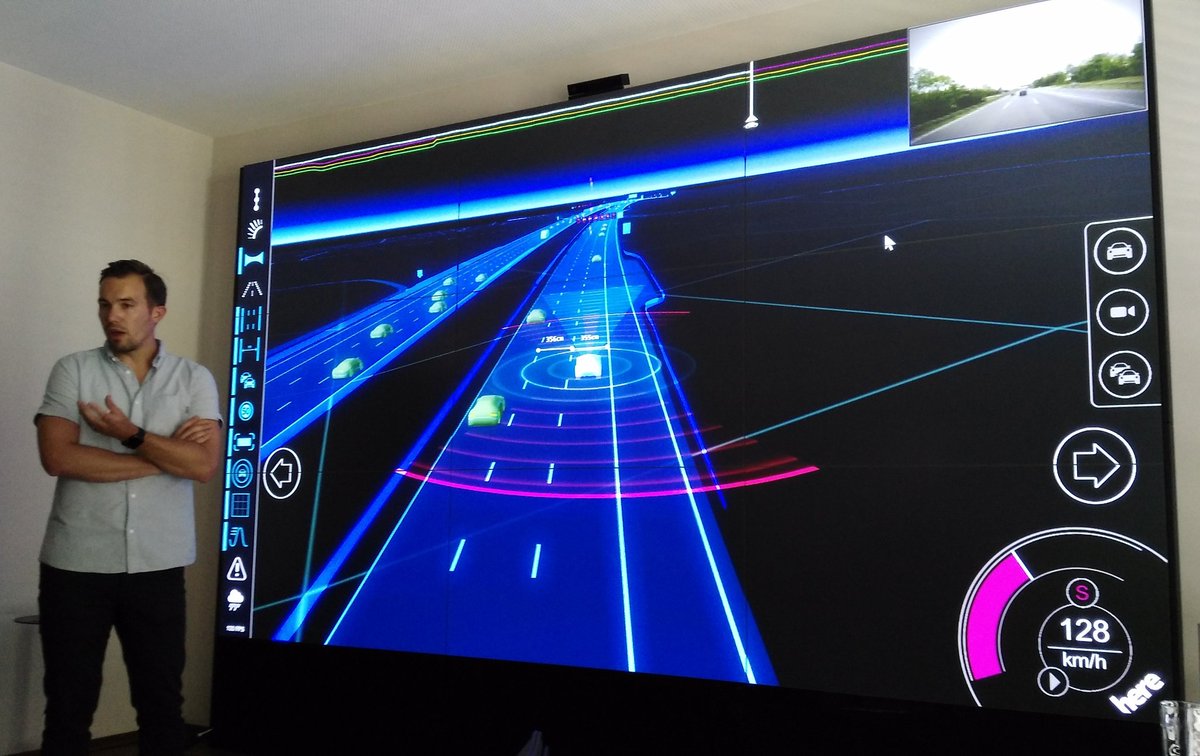
The data in these maps is highly compressed and delivered to cars, anywhere in the world, in cacheable 2km x 2km squares. (N.B. In one of the three pictures showing the maps of these datasets here, there is a mistake with the data shown. Can you see it? It’s obvious – once you’ve spotted it. No, it’s not that the cars on the wrong side of the road, as it’s showing a German autobhan rather than a British highway. Leave a comment if you find it!)
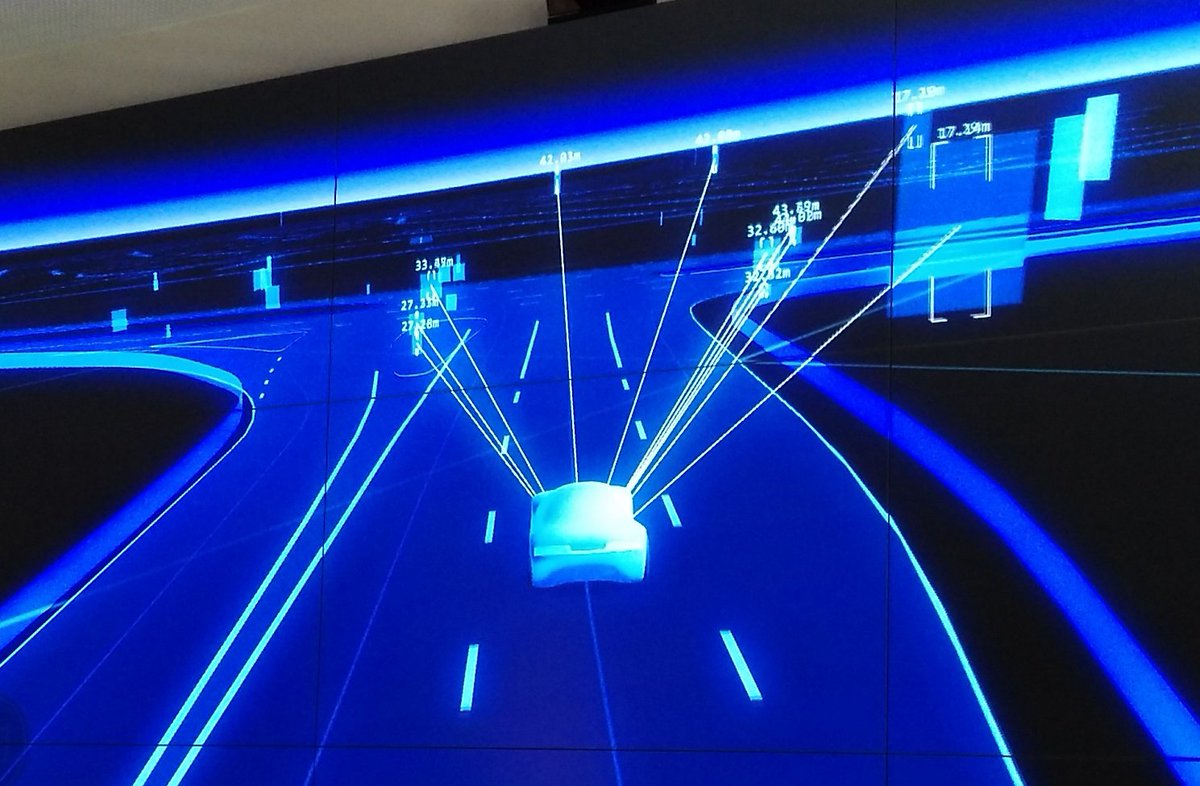
Spatial Data Visualisation
HERE also have some nice demo rigs to show their data in a context that is familiar to people, such as using a top-down projection on a 3D model city section, allowing data to be draped over the buildings and street structure:
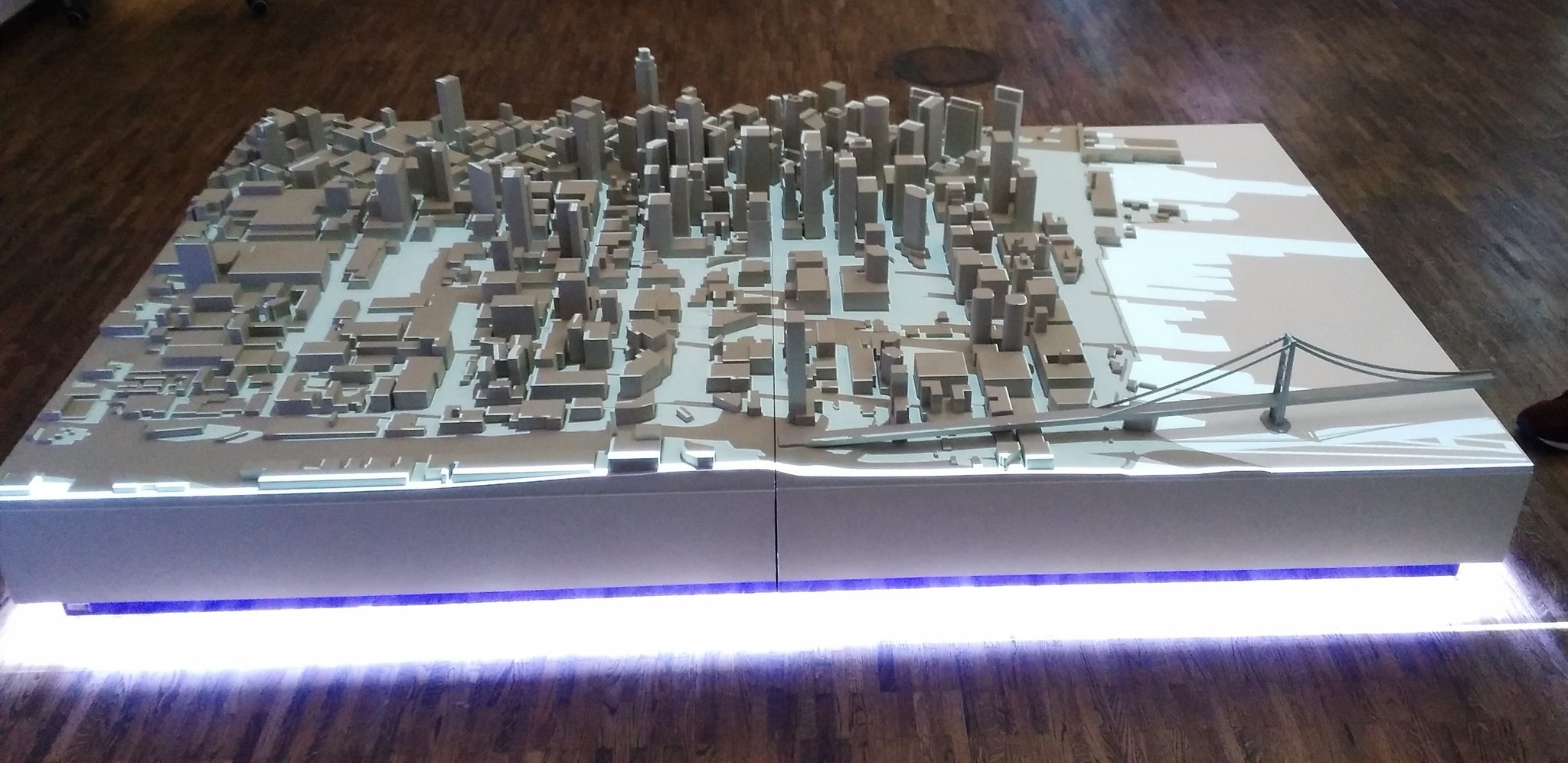
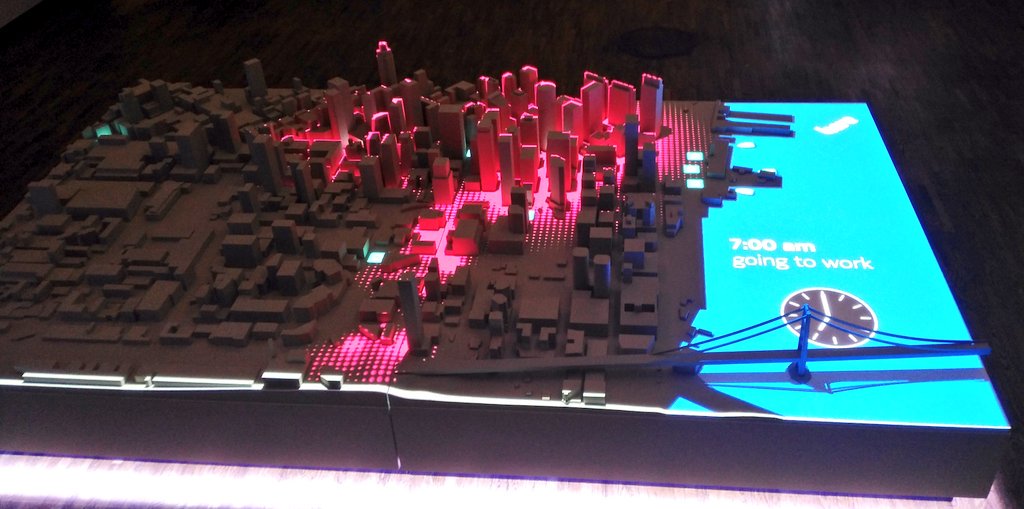
Transit Demand Modelling
We also saw a glimpse of a microsimulation-based travel demand model (TDM) for central Berlin, with what-if scenarios possible by placing various objects on the screen visualising the output of the model, such as a rain shower or closed road. The transport mode share will likely continue to adjust in large cities throughout the world, while the street network will often remain static, so such models (and associated visualisations) try and predict what will happen on the ground:
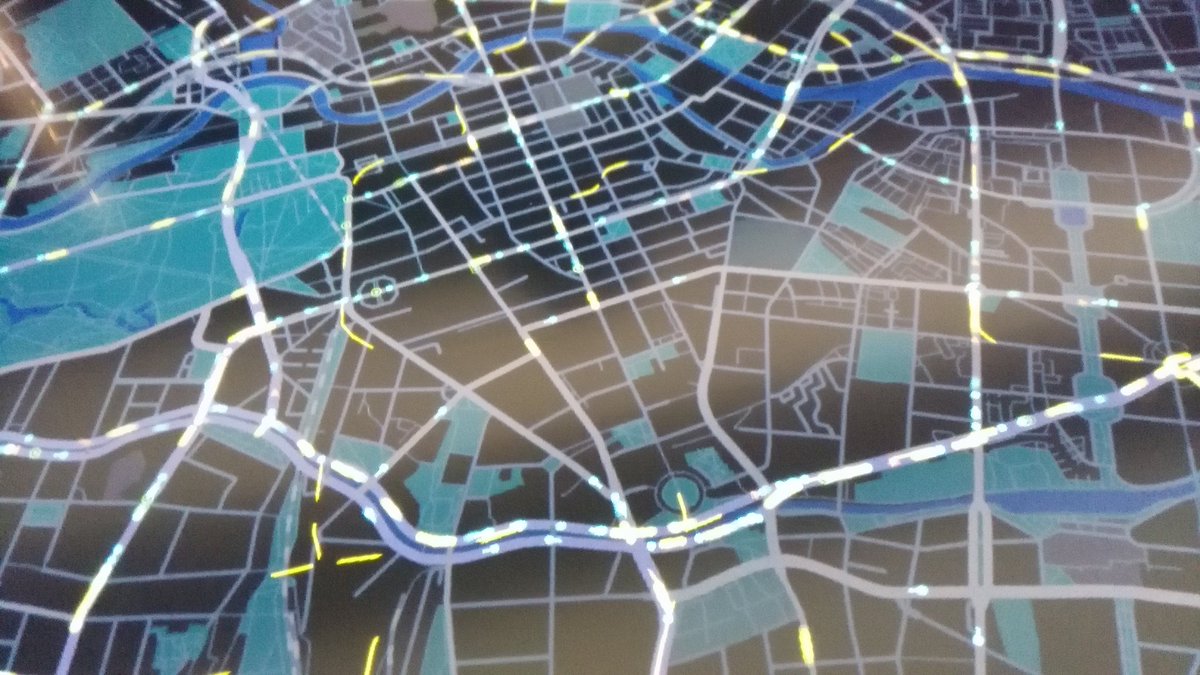
The other maps shown were in the user interface (i.e. dashboard/HUD) of a car test-rig, which is being used for UX/UI testing of autonomous/mixed-mode driving. I wrote about this in this previous blogpost.
HERE and the Future
Perhaps the most “exclusive” part of the day’s event was an hour long “fireside” chat with the new CEO of the company. As a relatively small group (there were around 10 of us)l, this was an excellent opportunity to grill the top-guy of one of the world’s three from-technology digital mapping providers (as opposed to from-GIS like ESRI or from-paper like the OS). Edzard Overbeek answered every question we threw at him efficiently. I quizzed him on whether indoor digital mapping, the “next frontier” identified by Google at least, will also be a priority for HERE given its new driving focus, to which Mr Overbeek was clear that, in order to be a serious player in the space it needs to be mapping everything, so that a single platform is available cross-use, i.e. if a customer journey ends with a walk through a department store, the platform needs to do the “last 100m” mapping too. It’s clear also that the HERE offline maps app will remain a key part of the company’s offering – not just to realise the value of their existing, long-built-up “consumer-grade” mapping, but to build the “HERE” brand to consumers. Ultimately though, their most important clients are the car companies – both the three that own the company but also others needing a “car mapping operating system”.