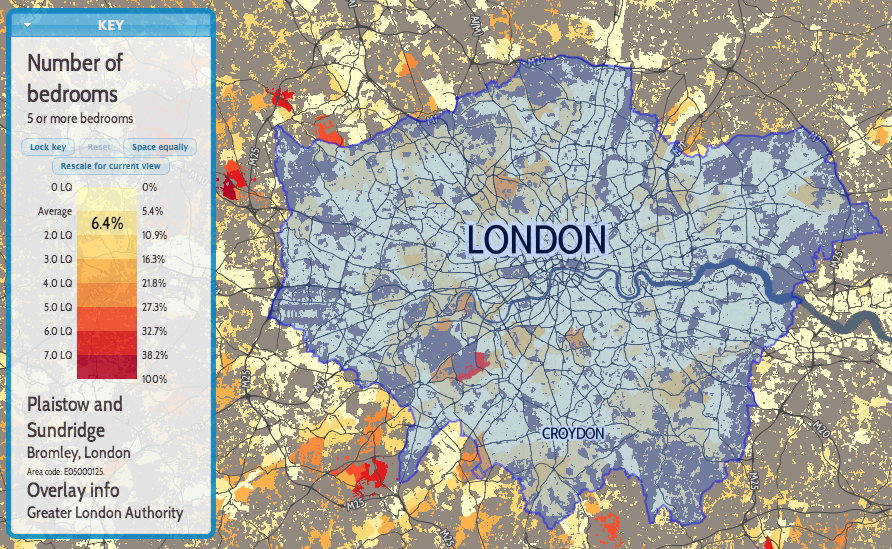
OpenLayers is a powerful web mapping API that many of my websites use to display full-page “slippy” maps. DataShine: Census has been upgraded to use OpenLayers 3. Previously it was powered by OpenLayers 2, so it doesn’t sound like a major change, but OL3 is a major rewrite and as such it was quite an effort to migrate to it. I’ve run into issues with OL3 before, many of which have since been resolved by the library authors or myself. I was a bit grumbly in that earlier blogpost for which I apologise! Now that I have fought through, the clouds have lifted.
Here are some notes on the upgrade including details on a couple of major new features afforded by the update.
New Features
Drag-and-drop shapes
One of the nicest new features of OL3 is drag-and-dropping of KMLs, GeoJSONs and other geo-data files onto the map (simple example). This adds the features pans and zooms the map to the appropriate area. This is likely most useful for showing political/administrative boundaries, allowing for easier visual comparisons. For example, download and drag this file onto DataShine to see the GLA boundary appear. New buttons at the bottom allow for removal or opacity variation of the overlay files. If the added features include a “name” tag this appears on the key on the left, as you “mouse over” them. I modified the simple example to keep track of files added in this way, in an ol.layer.Group, initially empty when added to the map during initialisation.
Nice printing
Another key feature of OL3 that I was keen to make use of is much better looking printing of the map. With the updated library, this required only a few tweaks to CSS. Choosing the “background colours” option when printing is recommended. Printing also hides a couple of the panels you see on the website.
Nice zooming
OL3 also has much smoother zooming, and nicer looking controls. Try moving the slider on the bottom right up and down, to see the smooth zooming effect. The scale control also changes smoothly. Finally, data attributes and credits are now contained in an expandable control on the bottom left.
A bonus update, unrelated to OL3, is that I’ve recreated the placename labels with the same font as the DataShine UI, Cabin Condensed. The previous font I was using was a bit ugly.
Major reworkings to move from OL2 to OL3
UTF Grids
With OpenLayers 3.1, that was released in December 2014, a major missing feature was added back in – support for UTF Grid tiles of metadata. I use this to display the census information about the current area as you “mouse over” it. The new implementation wasn’t quite the same as the old though and I’ve had to do a few tricks to get it working. First of all, the ol.source.TileUTFGrid that your UTF ol.layer.Tile uses expects a TileJSON file. This was a new format that I hadn’t come across before. It also, as far as I can tell, insists on requesting the file with a JSONP callback. The TileJSON file then contains another URL to the UTF Grid file, which OL3 also calls requiring a JSONP callback. I implemented both of these with PHP files that return the appropriate data (with appropriate filetype and compression headers), programmatically building “files” based on various parameters I’m sending though. The display procedure is also a little different, with a new ol.source.TileUTFGrid.forDataAtCoordinateAndResolution function needing to be utilised.
In my map initialisation function:
layerUTFData = new ol.layer.Tile({});
var handleUTFData = function(coordinate)
{
var viewResolution = olMap.getView().getResolution();
layerUTFData.getSource().forDataAtCoordinateAndResolution(coordinate, viewResolution, showUTFData);
}
$(olMap.getViewport()).on('mousemove', function(evt) {
var coordinate = olMap.getEventCoordinate(evt.originalEvent);
handleUTFData(coordinate);
});
In my layer change function:
layerUTFData.setSource(new ol.source.TileUTFGrid({
url: "http://datashine.org.uk/utf_tilejsonwrapper.php?json_name=" + jsonName
})
(where jsonName is how I’ve encoded the current census data being shown.)
Elsewhere:
var callback = function(data) { [show the data in the UI] }
In utf_tilejsonwrapper.php:
<?php
header('Content-Type: application/json');
$callback = $_GET['callback'];
$json_name = $_GET['json_name'];
echo $callback . "(";
echo "
{ 'grids' : ['http://datashine.org.uk/utf_tilefilewrapper.php?x={x}&y={y}&z={z}&json_name=$json_name'],
'tilejson' : '2.1.0', 'scheme' : 'xyz', 'tiles' : [''], 'version' : '1.0.0' }";
echo ')';
?>
(tilejson and tiles are the two mandatory parts of a TileJSON file.)
In utf_tilefilewrapper.php:
<?php
header('Content-Type: application/json');
$callback = $_GET['callback'];
$z = $_GET['z'];
$y = $_GET['y'];
$x = $_GET['x'];
$json_name = $_GET['json_name'];
echo $callback . "(";
echo file_get_contents("http://[URL to my UTF files or creator service]/$json_name/$z/$x/$y.json");
echo ')';
?>
Permalinks
The other change that required careful coding to recreate the functionality of OL2, was permalinks. The OL3 developers have stated that they consider permalinks to be the responsibility of the the application (e.g. DataShine) rather than the mapping API, and, to a large extent, I agree. However OL2 created permalinks in a particular way and it would be useful to include OL3 ones in the same format, so that external custom links to DataShine continue to work correctly. To do this, I had to mimic the old “layers”, “zoom”, “lat” and “lon” parameters that OL2’s permalink updated, and again work in my custom “table”, “col” and “ramp” ones.
Various listeners for events need to be added, and functions appended, for when the URL needs to be updated. Note that the “zoom ended” event has changed its name/location – unlike moveend (end of a pan) which sits on your ol.map, the old “zoomend” is now called change:resolution and sets on olMap.getView(). Incidentally, the appropriate mouseover event is in an OL3-created HTML element now – olMap.getViewport() – and is mousemove.
Using the permalink parameters (args):
if (args['layers']) {
var layers = args['layers'];
if (layers.substring(1, 2) == "F") {
layerBuildMask.setVisible(false);
}
[etc...]
}
[& similarly for the other args]
On map initialisation:
args = []; //Created this global variable elsewhere.
var hash = window.location.hash;
if (hash.length > 0) {
var elements = hash.split('&');
elements[0] = elements[0].substring(1); /* Remove the # */
for(var i = 0; i < elements.length; i++) {
var pair = elements[i].split('=');
args[pair[0]] = pair[1];
}
}
Whenever something happens that means the URL needs an update, call a function that includes this:
var layerString = "B"; //My old "base layer"
layerBuildMask.getVisible() ? layerString += "T" : layerString += "F";
[etc...]
layerString += "T"; //The UTF data layer.
[...]
var centre = ol.proj.transform(olMap.getView().getCenter(), "EPSG:3857", "EPSG:4326");
window.location.hash = "table=" + tableval + "&col=" + colval + "&ramp=" + colourRamp + "&layers=" + layerString + "&zoom=" + olMap.getView().getZoom() + "&lon=" + centre[0].toFixed(4) + "&lat=" + centre[1].toFixed(4);
}
Issues Remaining
There remains a big performance drop-off in panning when using DataShine on mobile phones and other small-screen devices. I have put in a workaround "viewport" meta-tag in the HTML which halves the UI size, and this makes panning work on an iPhone 4/4S, viewed horizontally, but as soon as the display is a bit bigger (e.g. iPhone 5 viewed horizontally) performance drops off a cliff. It's not a gradual thing, but a sudden decrease in update-speed as you pan around, from a few per second, to one every few seconds.
Additional Notes
Openlayers 3 is compatible with Proj4js version 2 only. Using this newer version requires a slightly different syntax when adding special projections. I use Proj4js to handle the Ordnance Survey GB projection (aka ESPG:27700), which is used for the postcode search, as I use a file derived from the Ordnance Survey's Code-Point Open product.
I had no problems with my existing JQuery/JQueryUI-based code, which powers much of the non-map part of the website, when doing the upgrade.
Remember to link in the new ol.css stylesheet, or controls will not display correctly. This was not needed for OL2.
OL3 is getting there. The biggest issue remains the sparsity of documentation available online - so I hope the above notes are helpful in the interim.
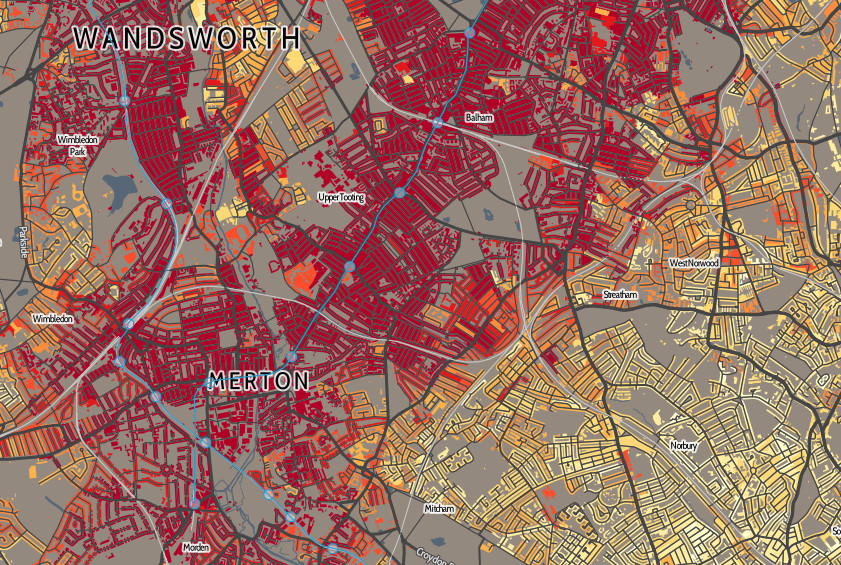
Above: GeoJSON-format datafiles for tube lines and stations (both in blue), added onto a DataShine map of commuters (% by tube) in south London.